안녕하세요! 저번 데이터 포털 관련 글에 이어서 조금 더 구체적인 데이터셋을 추천하고자 글을 남깁니다.
이미지 분류에 대한 데이터셋!! 지금 시작합니다~
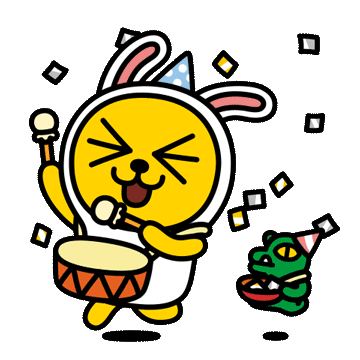
https://www.robots.ox.ac.uk/~vgg/data/fgvc-aircraft/#metric
FGVC-Aircraft
FGVC-Aircraft Benchmark Fine-Grained Visual Classification of Aircraft (FGVC-Aircraft) is a benchmark dataset for the fine grained visual categorization of aircraft. Please use the following citation when referring to this dataset: Fine-Grained Visual Clas
www.robots.ox.ac.uk
항공기 기종 분류를 위한 데이터셋입니다.
CNN 성능 테스트에 적합한 과제로 인정받아 현재 약 700개의 논문에서 활용되었습니다.
http://yann.lecun.com/exdb/mnist/
MNIST handwritten digit database, Yann LeCun, Corinna Cortes and Chris Burges
yann.lecun.com
기초적인 분류 문제에 활용되는 데이터입니다.
간단한 구조의 인공신경망으로도 분류가 가능한 데이터이므로, 자주 사용되고 있습니다.
Tensorflow, PyTorch등의 딥러닝 프레임워크에 내장되어 제공됩니다.
https://github.com/zalandoresearch/fashion-mnist
GitHub - zalandoresearch/fashion-mnist: A MNIST-like fashion product database. Benchmark
A MNIST-like fashion product database. Benchmark :point_down: - GitHub - zalandoresearch/fashion-mnist: A MNIST-like fashion product database. Benchmark
github.com
총 10개의 카테고리(티셔츠, 여성 정장 바지, 스웨터, 드레스, 코트, 샌들, 셔츠, 스니커즈, 가방, 발목 부츠)로 구성되어 있습니다.
Tensorflow, PyTorch등의 딥러닝 프레임워크에 내장되어 제공됩니다.
https://github.com/brendenlake/omniglot
GitHub - brendenlake/omniglot: Omniglot data set for one-shot learning
Omniglot data set for one-shot learning. Contribute to brendenlake/omniglot development by creating an account on GitHub.
github.com
영어를 포함한 50개의 알파벳에 대한 손 글씨 데이터셋을 제공합니다.
이미지 파일과 시간의 흐름에 따른 좌표 시퀀스를 제공합니다.
https://github.com/googlecreativelab/quickdraw-dataset
GitHub - googlecreativelab/quickdraw-dataset: Documentation on how to access and use the Quick, Draw! Dataset.
Documentation on how to access and use the Quick, Draw! Dataset. - GitHub - googlecreativelab/quickdraw-dataset: Documentation on how to access and use the Quick, Draw! Dataset.
github.com
Quick Draw! 게임을 통하여 구글이 수집한 손 그림 데이터셋으로, 총 345개의 카테고리에 대한 그림 데이터입니다.
https://github.com/visipedia/inat_comp
GitHub - visipedia/inat_comp: iNaturalist competition details
iNaturalist competition details. Contribute to visipedia/inat_comp development by creating an account on GitHub.
github.com
Competition에서 사용되는 자연 이미지 데이터셋으로, 굉장히 큰 규모의 데이터셋입니다.
https://mediatum.ub.tum.de/1454690
mediaTUM - Media and Publication Server
Author affiliation: Zhu, Xiaoxiang (TUM, DLR); Hu, Jingliang (DLR); Qiu, Chunping (TUM); Shi, Yilei (TUM); Bagheri, Hossein (TUM); Kang, Jian (TUM); Li, Hao (TUM); Mou, Lichao (TUM); Zhang, Guicheng (TUM); Häberle, Matthias (DLR); Han, Shiyao (TUM); Hua,
mediatum.ub.tum.de
인공위성으로 촬영된 지면의 사진과, 해당 지역이 어떤 종류의 땅인지 분류한 레이블입니다.
https://www.cs.toronto.edu/~kriz/cifar.html
CIFAR-10 and CIFAR-100 datasets
< Back to Alex Krizhevsky's home page The CIFAR-10 and CIFAR-100 are labeled subsets of the 80 million tiny images dataset. They were collected by Alex Krizhevsky, Vinod Nair, and Geoffrey Hinton. The CIFAR-10 dataset The CIFAR-10 dataset consists of 60000
www.cs.toronto.edu
각각 10개, 100개 카테고리에 대한 이미지를 제공합니다.
MNIST에 비해 분류 난이도가 높고, 의외로 분류가 잘되지 않는 데이터셋입니다.
https://www.vision.caltech.edu/datasets/cub_200_2011/
Perona Lab - CUB-200-2011
Caltech-UCSD Birds-200-2011 (CUB-200-2011) Warning: Images in this dataset overlap with images in ImageNet. Exercise caution when using networks pretrained with ImageNet (or any network pretrained with images from Flickr) as the test set of CUB may overlap
www.vision.caltech.edu
200종의 조류 사진입니다.
http://ufldl.stanford.edu/housenumbers/
The Street View House Numbers (SVHN) Dataset
SVHN is a real-world image dataset for developing machine learning and object recognition algorithms with minimal requirement on data preprocessing and formatting. It can be seen as similar in flavor to MNIST (e.g., the images are of small cropped digits),
ufldl.stanford.edu
원본 사이즈 이미지로, 길거리에서 촬영된 건물 주소 숫자 데이터입니다.
https://github.com/rgeirhos/texture-vs-shape
GitHub - rgeirhos/texture-vs-shape: Pre-trained models, data, code & materials from the paper "ImageNet-trained CNNs are biased
Pre-trained models, data, code & materials from the paper "ImageNet-trained CNNs are biased towards texture; increasing shape bias improves accuracy and robustness" (ICLR 2019 Oral) -...
github.com
텍스처와 모양이 서로 상충되는 이미지 데이터셋입니다.
https://github.com/google-research/si-score
GitHub - google-research/si-score
Contribute to google-research/si-score development by creating an account on GitHub.
github.com
배경 이미지 변화에도 강건하게 작동하는 분류 AI 개발을 위한 데이터셋입니다.
https://github.com/facebookresearch/co3d
GitHub - facebookresearch/co3d: Tooling for the Common Objects In 3D dataset.
Tooling for the Common Objects In 3D dataset. Contribute to facebookresearch/co3d development by creating an account on GitHub.
github.com
페이스북에서 공개한 데이터셋으로, 50개의 카테고리의 개체를 다각도에서 촬영한 데이터입니다.
이상으로 이미지 분류에 대한 데이터셋의 내용이였습니다.
'AI, 빅데이터 활용이 쉬워지는 142가지 데이터셋' 도서를 참고하여 작성하였습니다.
감사합니다!
'참고 > IT' 카테고리의 다른 글
데이터셋 - 자세인식 (1) | 2022.09.21 |
---|---|
데이터셋 - 안면인식 (0) | 2022.09.15 |
데이터셋 - 의미론적 연관성 (0) | 2022.09.15 |
데이터셋 - 탐지 및 표지 (0) | 2022.09.14 |
데이터 포털 (0) | 2022.09.13 |